Accidental falls are the second leading cause of injury-related death across the entire global population, and they take the top spot for people aged 65 and older. Centers for Disease Control and Prevention data show that fall death rates for that age group rose by about 30% between 2009 and 2018.
To combat this growing danger, tech developers have begun introducing products designed to either detect falls and automatically call for help or predict the likelihood that they’ll occur before they happen. A new artificial intelligence algorithm on the block is aiming to accomplish the latter goal but for a very specific patient group: lower limb amputees.
According to recent analyses, this group has an even higher risk of falling than the healthy geriatric population—with recorded incidence rates as high as 80% in some cases—but have long been left out of research into fall risk and its potential solutions.
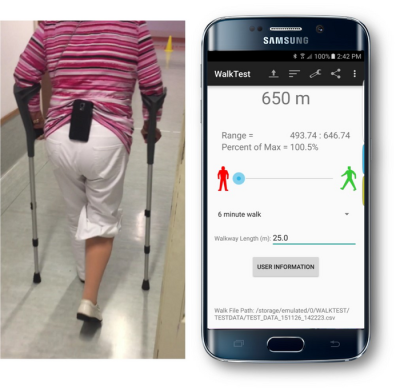
The new AI-powered system, which was developed by researchers from Canada and Slovenia, attempts to fill that gap. It specifically factored in the changes in gait that occur after lower limb amputations, resulting in an algorithm that can predict fall risk with similar accuracy to systems designed only with healthy older adults in mind.
As described in a study published this week in the journal PLOS Digital Health, the machine learning algorithm is embedded into a smartphone app. The smartphone is worn on a patient’s lower back throughout a standard six-minute walk test, known as a 6MWT.
After the walking portion is done, the AI gets to work. It was trained to automatically label each foot strike. Typically, this has to be done in a time-consuming manual process, since most other fall risk algorithms haven’t been programmed to analyze the “variability and instability” in the gaits of amputees, according to the study’s authors.
The study’s authors assigned the walk test to 80 participants, 27 of whom had experienced a fall within the preceding six months and thus were classified as having a higher risk of future falls. Each test was analyzed using both manual labeling of foot strikes and the new AI’s automated labeling.
Though the manual labeling was slightly more accurate in classifying the fall risk of all patients, the automated AI proved to be equally capable of identifying those with the higher fall risk, with both methods achieving a sensitivity of about 56%. Overall, accuracy of the manual labeling approach reached 80%, compared to slightly over 72% for the AI, which was set back by the six additional false positives it produced.
Altogether, the researchers concluded, those results suggest that the algorithm is a viable option to help calculate fall risk for lower limb amputees and could therefore “be integrated into a smartphone app to provide clinical assessment immediately after a 6MWT.”